Note
Click here to download the full example code
Adaptive / Reweighted LassoΒΆ
The adaptive or reweighted Lasso minimizes the non-convex L0.5 pseudo norm which is a better sparsity proxy function for the Basis Pursuit L0 norm. In practice, it promotes sparser coefficients with less amplitude bias. As with IndLasso, IndRewLasso is independent across tasks.
IndRewLasso solves the optimization problem:
(1 / (2 * n_samples)) * ||Y - XW||_Fro^2
+ sum_k alpha_k * ||W_k||_0.5
# Author: Hicham Janati (hicham.janati@inria.fr)
#
# License: BSD (3-clause)
import numpy as np
from matplotlib import pyplot as plt
from mutar import IndLasso, IndRewLasso
Generate multi-task data
rng = np.random.RandomState(42)
n_tasks, n_samples, n_features = 2, 20, 100
X = rng.randn(n_tasks, n_samples, n_features)
support = rng.rand(n_features, n_tasks) > 0.95
coef = support * rng.randn(n_features, n_tasks) * 5
y = np.array([x.dot(c) for x, c in zip(X, coef.T)])
# add noise
y += 0.5 * np.std(y) + rng.randn(n_tasks, n_samples)
alpha = 0.05 * np.ones(n_tasks)
Fit the independent Lasso
lasso = IndLasso(alpha=alpha)
lasso.fit(X, y)
Fit the independent reweighted Lasso
rewlasso = IndRewLasso(alpha=alpha)
rewlasso.fit(X, y)
Plot the supports of the true and obtained coefficients. Reweighting reduces the size of the active features and corrects the amplitudes of the relevant coefficients
coefs = [coef, lasso.coef_, rewlasso.coef_]
labels = ["Truth", "Lasso", "Re-Lasso"]
colors = ["k", "b", "r"]
lines = ["-", "--", "--"]
f, axes = plt.subplots(1, 2, figsize=(12, 4))
for c, ll, coefs, label in zip(colors, lines, coefs, labels):
for i, (ax, coef) in enumerate(zip(axes, coefs.T)):
# plot non-zero coefs only for clarity
xx = np.where(coef)[0]
yy = coef[xx]
ax.stem(xx, yy, label=label, linefmt=c + ll,
markerfmt=c + 'x', basefmt=c + ll)
ax.set_title("Coeffcient of task %d" % (i + 1))
ax.legend(loc=2, bbox_to_anchor=[1.01, 0.75])
plt.show()
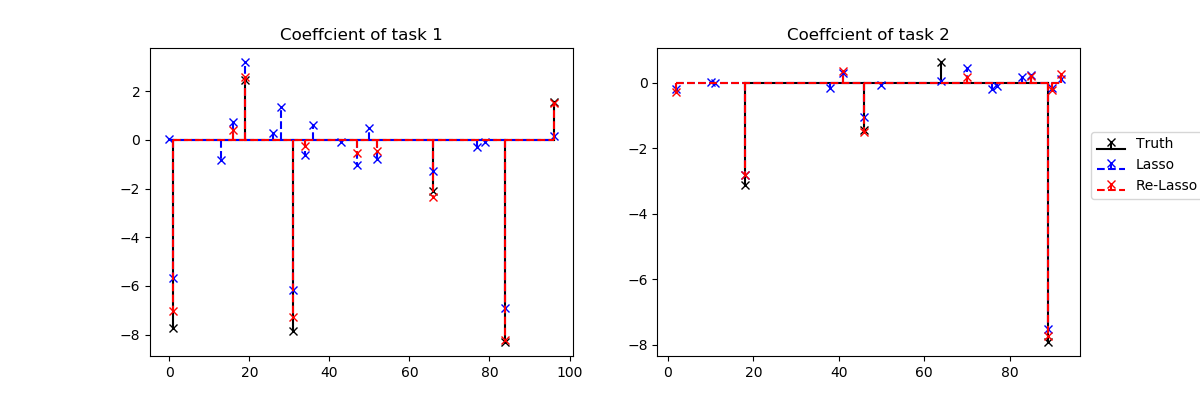
Estimated memory usage: 10 MB